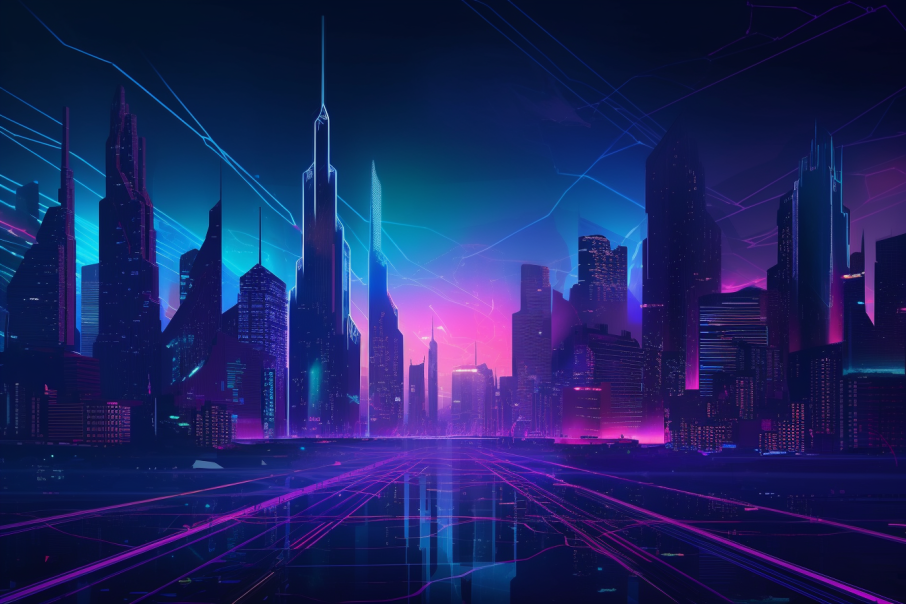
The Evolving Landscape of Data Engineering
Data engineering is becoming increasingly vital in today’s world. Businesses are leveraging powerful analytics tools to gain better insights into their customers’ behavior and make more informed decisions.
As such, staying up-to-date on the latest developments can give you an edge when it comes to managing data-driven projects. Having a solid foundation in this field can give you an edge when it comes to managing data-driven projects.
Therefore, I wanted to take a moment and chat about the ever-evolving landscape of data engineering. In this article, I’ll discuss some of the most important trends and technologies that are transforming the landscape of data engineering.
The Rise of DataOps: Revolutionizing Data Engineering Practices
As a data engineering analyst, I’m seeing huge changes in the way enterprises approach their data operations. The emergence of DataOps is revolutionizing how data engineers work. It facilitates better collaboration between stakeholders and gives us more control than ever before.
Automation optimization has become an essential part of our toolkit. We’re able to incorporate distributed architectures with greater ease and accuracy, helping organizations realize their goals faster.
Data virtualization also plays a key role in the evolution of data engineering practices. We no longer have to rely on traditional ETL processes; instead, self-service analytics allows us to access information quickly and easily without having to worry about making mistakes or violating any data governance regulations. This gives us unprecedented freedom and flexibility when it comes to working with big datasets.
The bottom line is that there’s no limit to what can be achieved with modern technologies for data engineering. From automating tedious tasks to providing secure access for remote teams, these tools are allowing us to continually improve our workflow efficiency while keeping our customers’ needs top-of-mind. It’s an exciting time for all of us who are involved in this field!
Harnessing the Power of Cloud-Based Data Engineering Solutions
Having explored the potential of DataOps in revolutionizing data engineering practices, we now turn to harnessing the power of cloud-based solutions. With increasingly large datasets and complex architectures, modern data engineers need more than traditional on-premise tools for near real-time insights.
As such, many organizations are turning to cloud computing technologies to deliver faster, more reliable, and cost-effective results:
- Scalable architectures: By leveraging distributed systems, container orchestration and serverless computing, data engineers can build highly scalable architectures. This can be done without compromising performance or stability.
- Cloud analytics: Big data platforms and machine learning algorithms enable data engineers to analyze larger volumes of data faster. This is especially true when combined with powerful visualization tools like Tableau or PowerBI. These tools allow them to easily share their findings with stakeholders across an organization.
- Data lakes & warehouses: Organizations looking to manage both structured and unstructured data can leverage cloud storage services. Amazon S3 or Google BigQuery are reliable services for their data lake needs. They can also take advantage of managed cloud databases like Azure SQL Server or Snowflake for warehousing applications.
All these components help create a unified platform where business users can access the data they need at any time. This allows business users to make informed decisions faster than ever before. By embracing this new wave of technology advancements, companies have been able to achieve greater levels of agility, scalability, and reliability, along with improved compliance and security standards.
In short, cloud-based solutions offer unprecedented opportunities for businesses seeking a competitive edge by unlocking actionable insights hidden within vast amounts of corporate knowledge stored in siloed systems around the globe.
The Increasing Importance of Data Privacy and Security in Data Engineering
Data security and privacy has become an increasingly important area of focus for data engineers in recent years. With the proliferation of new data privacy legislation around the world, organizations’ obligations to protect customer information increased. Organizations must be aware of their obligations to protect customer information or face costly penalties.
As a result, many are turning to cloud security solutions to protect their data. They are also turning to data anonymization techniques, access control policies, and encryption methods for data protection.
In my role as a data engineer, I have found it necessary to stay ahead of the curve when it comes to these emerging technologies and best practices. For instance, using cloud storage allows us to quickly deploy applications without having to worry about setting up complex infrastructure. Cloud storage also provides robust security controls such as authentication protocols and granular access control policies.
Additionally, by incorporating advanced encryption methods, we can secure our databases while still allowing users quick access to the needed information.
Developing a deep understanding of how different technologies work together is key in order to properly assess risks and vulnerabilities. In particular, data anonymization techniques like masking certain pieces of personally identifiable information are becoming more commonplace. This is due to increased awareness from both customers and businesses alike regarding the need for better online privacy protections.
Embracing Machine Learning and AI Integration in Data Engineering
As data engineers, it’s essential that we stay ahead of the curve and embrace new trends and technologies. In particular, machine learning (ML) and artificial intelligence (AI) have become increasingly important in recent years. This enables us to make more informed decisions faster than ever before.
With predictive analytics, automation tools, and self-service analytics solutions at our fingertips, we’re able to unlock valuable insights from vast amounts of data with greater accuracy and efficiency.
However, while ML and AI can be major assets when used correctly, they do present certain scalability challenges. Chief among those is the need for a robust data governance policy — one that establishes clear security protocols while providing users with access to their desired information quickly and reliably.
This requires an understanding of how personalization works within each system as well as how user preferences are stored safely so that only authorized personnel have access.
By leveraging these advanced technologies responsibly, data engineers can ensure better results without compromising on security or privacy standards.
We must continue to strike a balance between advancing our skillset while being mindful of potential pitfalls — all with the goal of creating reliable systems that offer real value to our customers.
The Growing Demand for Real-Time Data Processing and Streaming Technologies
Data engineering has been gaining traction in the past few years due to its ability to help organizations leverage data. As a result, there is an ever-increasing demand for real-time data processing and streaming technologies.
The most popular platforms used by organizations are those which incorporate distributed computing capabilities in order to process large volumes of data quickly and efficiently. This helps enable predictive analytics that provide better insight into customer behavior or market trends. This gives businesses more control over their operations.
Furthermore, unified governance structures allow for automated pipelines, reducing manual effort and the risk of errors significantly. Self-service analytics tools also make it easier for non-technical users to access relevant information. This allows them to work independently of IT departments for assistance.
These benefits have driven the need for robust technology solutions that focus on scalability and reliability as core tenets when delivering real-time data processing capabilities. By leveraging these technologies, companies are able to stay competitive while at the same time driving greater value from their investments in data engineering projects.
Conclusion
Data engineering is transforming at an impressive rate. Over the past decade, we have seen a dramatic shift towards cloud-based solutions, machine learning, and AI integration, data privacy, real-time streaming technologies, and more.
This evolution has only been accelerated by the pandemic of 2020, which saw a staggering 61% increase in global spending on IT infrastructure services to ensure business continuity (Gartner).
As data engineers, it’s up to us to stay up-to-date with these trends and shape our practice accordingly – for if there’s one thing that hasn’t changed since day one of this profession, it’s that staying ahead of the curve means success!